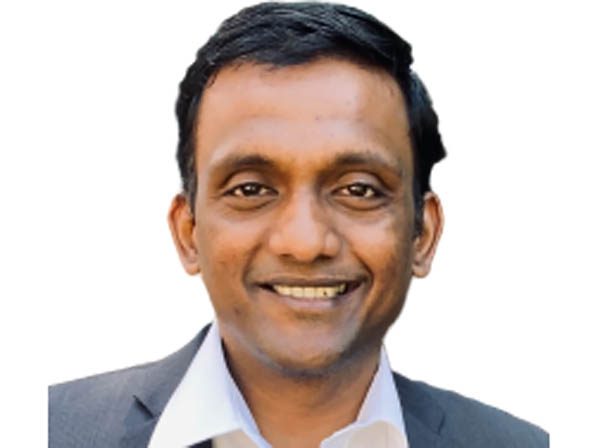
The sudden advent of large language model (LLM) AI tools, such as ChatGPT, Duet AI for Google Cloud, and Microsoft 365 Copilot, is opening new frontiers in AI-generated content and solutions. But the widespread harnessing of these tools will also soon create an epic flood of content based on unstructured data – representing an unprecedented level of risk to Data Governance.
In this post, I will explore the five most critical Data Governance challenges presented by LLM AI tools and provide helpful tips for addressing them.
Data Privacy Concerns
LLM AI tools can inadvertently expose sensitive or private information, jeopardizing individual privacy rights and breaching data protection regulations.
Be sure to take stock of the types of data being fed into the LLM AI tools and assess their sensitivity. Before training the models, apply techniques such as data anonymization or masking to protect personally identifiable information. Finally, implement strict access controls to limit who can retrieve and interact with the AI-generated content, ensuring that only authorized individuals can access sensitive data.
Data Security
The sheer volume of content generated by LLM AI tools increases the risk of data breaches and unauthorized access to valuable information.
It’s essential to utilize encryption techniques to protect data while it’s being transferred and stored. Stay proactive by implementing the latest security patches and protocols to mitigate vulnerabilities. Regularly assess and audit the security measures around LLM AI tools to identify and address any potential weaknesses.
Compliance Challenges
LLM AI tools can create compliance challenges as they generate content without proper consideration for regulatory requirements, leading to potential legal and ethical implications.
Establishing clear policies that outline how data should be handled, ensuring alignment with relevant regulations and ethical guidelines, is fundamental. It is also wise to incorporate compliance considerations when training LLM AI models by utilizing datasets that are representative of the organization’s compliance requirements. And make sure to regularly monitor the content generated by LLM AI tools to identify any compliance deviations and take corrective action promptly.
Transparency
LLM AI tools operate as black boxes, making it challenging to understand how they generate content, raising concerns about biases, fairness, and accountability.
It is key to incorporate explainability methods to shed light on how LLM AI tools make decisions, providing insights into the underlying processes. Also, regularly evaluate the content generated by these tools for potential biases and take corrective action to ensure fairness and inclusivity, while encouraging open communication and documentation regarding the use of the tools – ensuring stakeholders are aware of their limitations and potential biases.
Bias and Ethics
Since models are trained to behave and reason using massive troves of existing data – usually from historical interactions – models will start mimicking the behaviors in that training data.
For instance, if our past loan approvals utilized race or income or ethnicity, using that as training data will simply teach the model to profile and become potentially racist.
Working with LLM models requires extra caution to identify the presence of potential profiling in data attributes in training data. Care must also be put into reviewing responses for inadvertent biased or unethical behaviors expressed by the models.
Conclusion
The rapid adoption of LLM AI tools brings both excitement and challenges for Data Governance. Embracing a proactive and holistic approach to Data Governance will help mitigate the potential pitfalls and unlock the full potential of these tools while safeguarding privacy, security, and regulatory compliance.
Let’s embrace this new era of AI responsibly and shape a future where ethical Data Governance remains paramount.